
Differential Privacy (DP) – In-Depth Technical Description
Definition:
Differential Privacy (DP) is a rigorous mathematical framework designed to quantify and enforce the privacy of individual data points within statistical databases. The core idea is to ensure that the inclusion or exclusion of a single record in a dataset does not significantly affect the output of any analysis, thereby minimizing the risk of identifying specific individuals. This is achieved by introducing controlled randomness (noise) into computations or queries.
---
Mathematical Foundation:
A randomized algorithm is said to satisfy -differential privacy if, for any two datasets and differing by a single record, and for all possible outputs :
Pr[M(D) \in S] \leq e^\epsilon \cdot Pr[M(D') \in S]
: Privacy budget – a non-negative parameter controlling the privacy-utility trade-off. Smaller values indicate stronger privacy guarantees.
and : Neighboring datasets differing by only one element.
: Subset of possible outputs of the mechanism.
Intuitively, this inequality ensures that the probability of obtaining a particular output remains almost the same regardless of whether any single individual's data is present, limiting the amount of information leaked.
---
Mechanisms of Noise Addition:
To achieve differential privacy, noise is introduced to query results or model parameters through various mechanisms. Common techniques include:
1. Laplace Mechanism:
Noise drawn from the Laplace distribution is added to the output of a query. The scale of the noise is proportional to the sensitivity of the function.
M(D) = f(D) + Lap(\frac{\Delta f}{\epsilon})
\Delta f = \max_{D,D'} | f(D) - f(D') |
2. Gaussian Mechanism:
Applies Gaussian noise for scenarios where stronger privacy guarantees (such as ()-differential privacy) are required.
M(D) = f(D) + N(0, \sigma^2)
3. Exponential Mechanism:
Used for non-numeric outputs by selecting an outcome with a probability exponentially proportional to a scoring function.
---
Sensitivity Analysis:
Sensitivity measures how much the output of a function can change when a single data point is modified. Lower sensitivity reduces the noise required for achieving DP, improving utility while maintaining privacy.
Global Sensitivity: Upper bound on the output variation across all neighboring datasets.
Local Sensitivity: Sensitivity specific to a particular dataset.
---
Composition Theorems:
Sequential Composition: The overall privacy loss of differentially private mechanisms applied sequentially to the same dataset is bounded by the sum of their privacy parameters.
\epsilon_{total} = \sum_{i=1}^{k} \epsilon_i
---
Real-World Applications:
Google RAPPOR (Randomized Aggregatable Privacy-Preserving Ordinal Response): Differentially private data collection from user devices.
Apple's Differential Privacy Framework: Used to aggregate user behavior data without compromising individual privacy.
U.S. Census Bureau: Implements DP to protect the confidentiality of census data while releasing aggregate statistics.
---
Challenges and Considerations:
Privacy-Utility Trade-off: Higher noise levels provide stronger privacy but can degrade the accuracy of results.
Calibrating : Selecting appropriate values of is complex and context-dependent. A balance must be struck between usability and privacy.
Longitudinal Data: Applying DP to datasets evolving over time introduces complexities in ensuring privacy across multiple time periods.
Correlated Data: Traditional DP assumes independent records; correlations can undermine privacy guarantees.
---
Advanced Concepts:
Local Differential Privacy (LDP): Noise is added directly on user devices before data collection, ensuring privacy even from data aggregators.
Adaptive Differential Privacy: Privacy guarantees are preserved even if the adversary selects queries adaptively based on prior outputs.
Zero-Concentrated Differential Privacy (zCDP): A refinement providing tighter bounds on privacy loss by leveraging Rényi divergence, reducing unnecessary noise in practical applications.
---
Conclusion:
Differential Privacy represents a cornerstone of modern data privacy techniques, providing formal guarantees against re-identification and adversarial inference. As data-driven technologies continue to expand, the adoption of DP frameworks is essential to balance innovation with privacy, fostering trust and compliance in sensitive data environments.
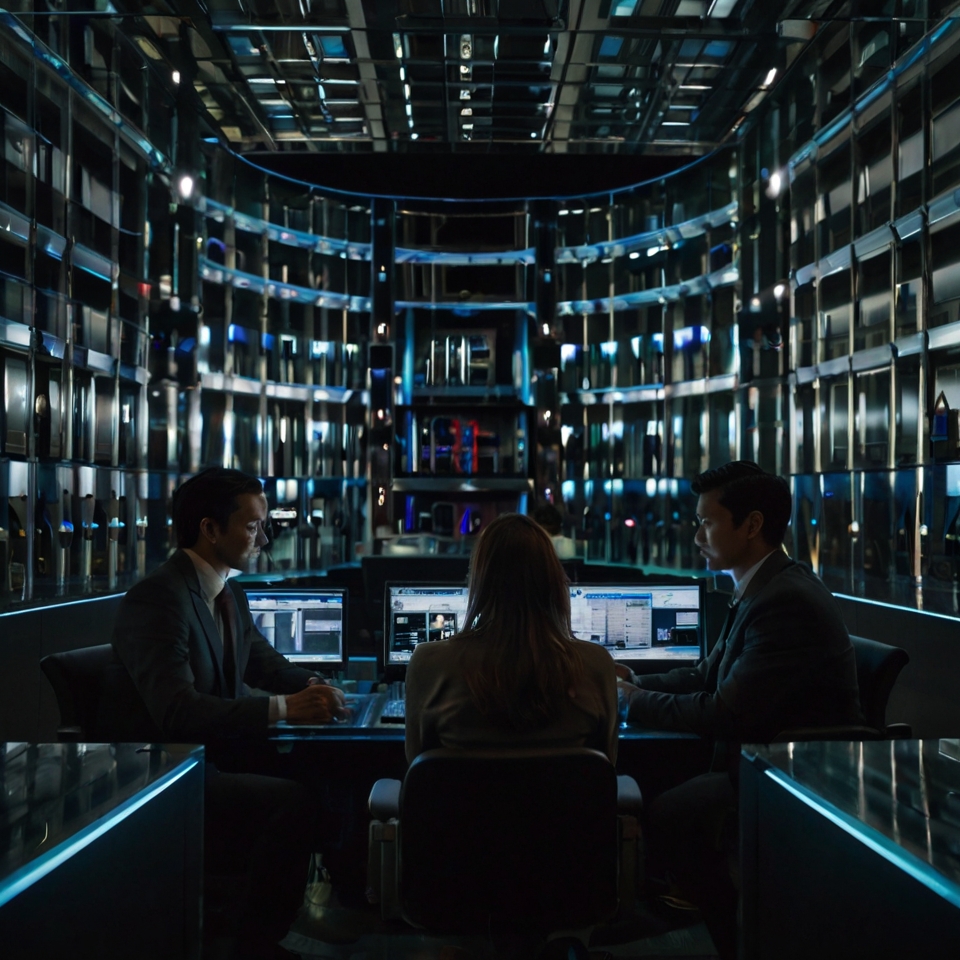